AI and drug discovery
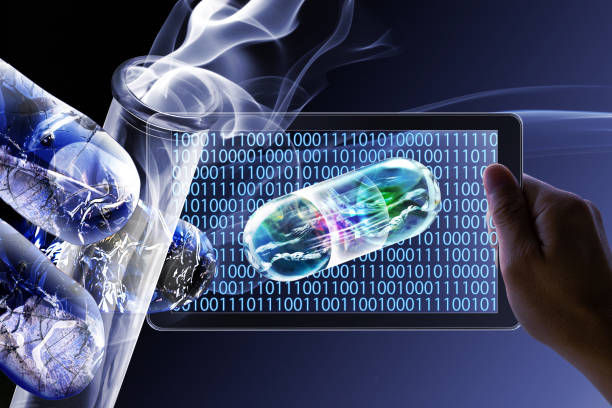
In recent times, AI is emerging with new technologies in all fields. The reason AI is becoming popular is that it minimizes human efforts and completes the work with the help of machines efficiently.
Recent studies have shown the importance of AI in oral healthcare too.
Please read this article: AI for sustainable oral healthcare
Keywords: AI, pharmaceutical sector, E-VAI, drug, linear discriminant analysis (LDA), support vector machines (SVMs), random forest (RF), decision trees, NOVARTIS
Over the past years, the pharmaceutical sector has experienced vast digitalization. To achieve digitalization, the technology needs to be able to deal with complex clinical problems. This motivates AI, as it is a great way to find a solution to all complex problems using advanced technology.
The components of AI are shown in the diagram below:
E-VAI is an AI platform developed by Eularis. It makes use of ML algorithms along with an easy-to-use user interface for helping in pharmaceutical sales. It helps marketing executives for dealing with the allocation of resources for maximum sales and investing in the right place.
AI helps the pharmaceutical sector in the following ways:
- It can aid rational drug design.
- Assist in decision making such as determining the right therapy for a patient, including personalized medicines.
- Managing the clinical data generated and aiming to use it for future drug development.
- Ensure QA with aid of ELN and other techniques.
- Automated manufacturing.
- Understanding critical process parameters.
- Market prediction and Analysis.
- Product costing.
- Ensuring in-process specification compliance.
- Drug-protein interaction.
- Bioactivity prediction.
Generally, the development of drugs is in huge amounts followed by a vast chemical space and a large number of drug molecules. Due to the lack of advanced technologies, the development process results to be time-consuming and expensive. This issue can be resolved using AI. AI can provide a quicker validation of the drug.
QSAR modeling tools have been utilized for the potential drug candidates. AI-based QSAR approaches include:
- linear discriminant analysis (LDA)
- support vector machines (SVMs)
- random forest (RF)
- decision trees
Current market scenario w.r.t. AI:
The AI market increased from US$200 million in 2015 to US$700 million in 2018. It is also expected to increase to $5 billion by 2024. A 40% growth is projected from 2017 to 2024. This indicates that AI will expand more in near future. Also, various pharmaceutical companies are collaborating with AI companies for the development of essential healthcare tools. For example, Pharmaceutical organization NOVARTIS has collaborated with Microsoft for discrete reasons such as researching on cell and gene-based therapies, Optimization of the same, etc. AI-based solutions in the pharmaceutical sector, with an investment exceeding US$7.20 billion. Also, there were 300+ deals between 2013 and 2018 by the pharmaceutical industries.
Drawback:
The only drawback of using AI-based technologies is generating unemployment. The aim of AI is not to replace humans, but to reduce human efforts. Thus, it reduces the requirement of humans for work.
Conclusion:
The traditional way of developing drugs is quite time-consuming and expensive. But the use of AI will not only help in dealing with these issues but also help in providing an efficient product to the market. As it will provide a cost-effective product, utilization will rise too. AI will also ensure the safety of the product by providing optimization.
Story Source:
Materials provided by National Library of Medicine. The original text of this story is licensed under a Creative Commons License. Note: Content may be edited for style and length.
Journal Reference:
0 Comments