Human-Machine Collaboration in Intelligence Analysis: An Expert Evaluation
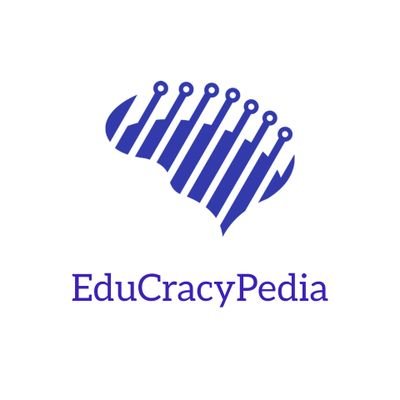
An intelligence analyst’s job is to construct coherent hypotheses despite significant gaps and inconsistencies in gathering evidence, and present them clearly to decision-makers to inform their interventions.
Current automated systems to support the day-to-day practice of analysts are (almost) exclusively focused on two aspects of the problem. The first is data collection, aggregation, and visualization. Such tools help analysts collate, inspect and interact with a large dataset, and support the identification of relationships, for example through link analysis. Recently, crowdsourcing tools that enable the public to contribute information have also been introduced to integrate more traditional intelligence collection approaches. The second problem on which tools focus involves listing and weighing up alternative hypotheses, through automated analysis of competing hypotheses. This analysis requires that all alternative hypotheses be identified from available evidence, and, if aided by automated inferential reasoners such as Bayesian networks, the tools also require that each (aggregated) piece of evidence is given a weight or a degree of certainty.
We observe, however, that there is a gap in technology that supports the process that analysts perform after inspecting the data, and before the identification of hypotheses. The task of the analyst here consistently involves the structuring of evidence to select plausible hypotheses. This is currently done manually, supported only by generic spreadsheets and text-processing tools. The challenge we seek to address in this research is to understand how automated reasoning can best complement human expertise in this evidential reasoning process.
Experienced analysts currently identify plausible hypotheses using a combination of manual approaches to assess available evidence, establish what information is credible, and understand what additional evidence may be required or what questions to ask to determine plausibility. This activity may be time critical to enable effective situational understanding, which poses significant challenges for individual analysts. The volume and variety of information that analysts must consider are significant, and, evidence may be unreliable or conflicting, with important information missing. Collaboration may be used to provide peer review, share the burden of analysis, and help in the validation of conclusions. Such collaboration, however, requires analysts to work with a common model and a consistent worldview, which is hard to achieve in the real world.
When data is diverse and comes from different sources, analysts must reason about the reliability of the evidence leading to claims from information such as how, where, when, and by whom the evidence was gathered and analyzed. Cognitive biases may inadvertently be introduced in the process, preventing an analyst from drawing accurate conclusions. This process of interpreting evidence relies heavily on the expertise and training of analysts, and there is a distinct lack of methods to ease the high cognitive burden involved in forming hypotheses. Furthermore, there is a general lack of understanding of how the hypothesis formation process works, as it is not normally recorded, making it difficult for senior analysts to pass on their analytical skills to trainees. The analytical process is also resistant to automation due to the significant knowledge engineering effort required to process data and express reasoning patterns.
In this paper, we have illustrated how novel AI methods, based on a combination of argumentation theory, crowdsourcing, and provenance reasoning can contribute to improved performance in intelligence analysis. While existing systems care mostly about information presentation and collection we co-designed our software tool — CISpaces — with intelligence analysts to focus on the sensemaking activities around forming hypotheses from available evidence using patterns of defeasible inferences, or argumentation schemes. Our formal evaluation of CISpaces using the Technology Acceptance Model (TAM) provides evidence that intelligence analysts benefit from the support they receive from the tool in their sensemaking activities.
The contributions of this paper are thus manyfold:
- •
In 3rd section, we provide a blueprint for further co-design of artificial intelligence-driven tools, by showing how to govern the process for a successful outcome.
- •
In Section 4, we expand on our preliminary conference paper to illustrate the delicate interconnection between the various artificial intelligence techniques utilized and extended to achieve the co-designed objectives. In particular:
- •
we advance the engineering of argumentation-based reasoning to identify plausible hypotheses as sets of acceptable arguments;
- •
we show how to argue with, and about, crowd-sourced information pre-analyzed using Bayesian analysis;
- •
we embed provenance analysis in the argumentative process to establish the credibility of hypotheses.
- •
In Section 5 we provide empirical evidence that intelligence analysts benefit from the unique mixture of formal argumentation theory, crowdsourcing support, and provenance recording provided in spaces, for the first time, using the Technology Acceptance Model (TAM) in an argumentation-based system.
Our results suggest that the novel, principled AI methods implemented in CISpaces may advance performance in intelligence analysis. Despite having designed CISpaces as a basic research prototype (TRL 3), during their evaluation, analysts benchmarked the quality of its features against commercial systems they use every day: we compare them in Section 6.
We collected evidence suggesting that the AI methods implemented in CISpaces can have a behavioral effect on end users' intention to adopt spaces. The analysts’ evaluation highlights drawbacks in CISpaces that predominantly result from the interface between the tool and data sources and aspects of the user interface (rather than the underlying AI methods). We, therefore, conclude that for successful adoption by the intelligence analysis community, CISpaces will need data integration with existing organizational standards both for the input and the output of information. These and other engineering and usability aspects, while being essential for commercialization, are beyond the scope of this paper.
Challenges of Intelligence Analysis:
Intelligence analysis is the application of individual and collective cognitive methods to evaluate, integrate, and interpret information about situations and events, aiming to provide a warning regarding potential threats or to identify opportunities. Various types of intelligence can be distinguished based on the source. HUMINT (human intelligence), for example, is intelligence gathered from human sources. IMINT (imagery intelligence) is derived from image or video sources. OSINT (open source intelligence) is acquired from sources such as social media and more recent types of intelligence include for example crowdsourced intelligence, made up of structured information acquired from or volunteered by the general public. Analysts often specialize in a specific type of intelligence and may be focused on particular objectives (e.g., tracking activities of a criminal organization). In the military context, strategic analysts focus on studying the long-term objectives and intentions of foreign actors, while operational and tactical analysts are focused on supporting specific actions or providing timely responses to emerging situations.
model of intelligence analysis is one of the most influential in training and practice. It consists of two high-level iterative loops: foraging for information which is collected, filtered, and collated into evidence files; and sensemaking, where the evidence files are interpreted through logical reasoning by drawing inferences and identifying hypotheses, which are then brought together to form a coherent explanation of the situation. A sketch of this process is shown within the box at the top of below figure no.1. The top row represents general features that characterize or influence the reasoning process during analysis. The other rows in this figure represent concepts related to different dimensions of intelligence analysis corresponding to a specific phase of analysis represented by the curly bracket in the column. More generally, this figure provides a reference for the components and challenges which inform the remainder of our discussion and are ordered according to the topics covered in this Section.
Story Source:
Materials provided by Intelligent Systems with Applications. The original text of this story is licensed under a Creative Commons License. Note: Content may be edited for style and length.
Journal Reference: Science direct
0 Comments