Model Reveals Optimal Learning Rates for Organisms in Changing Environments
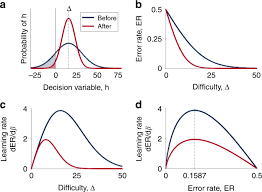
Researchers from the Complexity Science Hub and Santa Fe Institute have developed a groundbreaking model to determine the ideal learning rate for organisms in response to environmental changes. Their findings suggest that an organism's optimal pace of learning is closely linked to both the rate of environmental change and its life cycle.
As the world constantly evolves, every organism—from microscopic bacteria to majestic blue whales—faces the challenge of adapting to shifting surroundings. Animals must learn to locate nourishing food sources that may vary with the seasons, making efficient learning crucial for survival. However, the process of learning requires both time and energy; an organism that learns too slowly risks falling behind, while one that learns too quickly may waste resources tracking insignificant changes.
The researchers' mathematical model provides a quantitative approach to understanding the optimal learning pace. "The key insight is that the ideal learning rate increases in a consistent manner, regardless of how quickly the environment changes or how the organism interacts with it. This suggests a universal principle applicable across diverse ecosystems," explains CSH PostDoc Eddie Lee.
The model envisions an environment that transitions between various states, such as wet and dry seasons, at a defined tempo. The organism perceives its surroundings and maintains a memory of past states, but older memories lose relevance over time, influenced by the organism's learning timescale.
Learning at the Square Root of Change
What is the ideal learning timescale for optimizing adaptation? The model proposes a universal law: the learning timescale should be proportional to the square root of the environmental timescale. For instance, if environmental changes occur at half the speed, the organism's learning rate should decrease by a factor of 1.4 (the square root of 2). This relationship indicates a balance between learning too quickly and too slowly, while also suggesting diminishing returns on longer memory retention.
Additionally, the model explores organisms capable of actively shaping their environment, known as niche construction. Lee notes that if an organism can stabilize its environment, it gains an evolutionary advantage—provided it can monopolize the benefits. For example, beavers create stable habitats by building dams, securing a consistent food supply and protection from predators. However, this advantage can be undermined if other species exploit the resources of these constructed niches.
Metabolic Overhead for Large Animals
The researchers also examined how learning abilities relate to metabolic costs—the energy requirements of an organism. They predict that for small, short-lived creatures like insects, the energy costs associated with learning and memory are significant. Conversely, for larger, longer-lived animals like mammals, the metabolic demands overshadow the costs of learning. This implies that smaller organisms exhibit finely-tuned memory adaptations, while larger animals, such as elephants, may retain information for longer but are influenced by factors beyond learning, such as social group dynamics.
The findings highlight that while small organisms have adapted to their environments with precision, larger species balance their memory retention against other survival needs. The new model provides a quantitative framework for understanding how organisms navigate the complexities of learning and survival in a dynamic world, suggesting an optimal adaptation rate that aligns with environmental change and lifespan across various life forms, from microbes to humans.
Story Source:
Materials provided by Complexity Science Hub. The original text of this story is licensed under a Creative Commons License. Note: Content may be edited for style and length.
Journal Reference:
- Edward D. Lee, Jessica C. Flack, David C. Krakauer. Constructing stability: optimal learning in noisy ecological niches. Proceedings of the Royal Society B: Biological Sciences, 2024; 291 (2033) DOI: 10.1098/rspb.2024.1606
0 Comments