New computing architecture - Deep learning with light
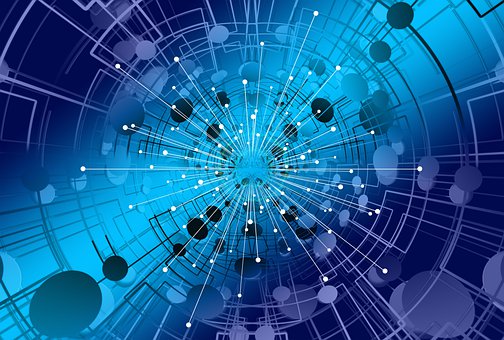
Whenever we ask a particular gadget to perform a certain task it takes several seconds to show the outcome. The reason behind this latency is that the devices don't have high memory power to quickly understand the requirements of the user. Also, the distance may be one of the factors. The device is placed quite a distance away. After the request, the answer is computed and then sent back to the device.
Keywords: Latency, fiber optic, light waves, computations, smart transceiver, weights, silicon photonics
Now, researchers have discovered a new way for computations on these devices. This new way surely helps in reducing the latency in the devices. The idea is to shift the memory-intensive steps of a model to a central server where components are encoded onto light waves. These waves are transmitted using fiber optics to a device. It provides a way for huge data to be sent lightning-fast over a network. The receiver then assists a simple optical device in performing computations. It rapidly performs calculations using components of the model that are carried by light waves.
The neural network architecture that is developed works on the idea of storing the data in a central server. This central server is connected to a smart transceiver. A smart transceiver refers to a small chip that uses silicon photonics technology for transmitting and receiving weights.
It receives weights in the form of electrical signals that are in the form of bits(1s and 0s). The transceiver converts them by using the switching lasers method i.e. a laser is turned on for a 1 and it is turned off for a 0. It combines these light waves and transfers them by making use of fiber optics.
This method comparatively provides great efficiency. It also provides better security. This method has various real-time applications:
1. It can enable a self-driving car that can make decisions using less percentage of energy.
2. It can create a latency-free conversation environment between the user and a smart home device.
3. Can be used for Image classification in space missions.
Senior author Dirk Englund, an associate professor in the Department of Electrical Engineering and Computer Science (EECS) and member of the MIT Research Laboratory of Electronics said, "Every time you want to run a neural network, you have to run the program, and how fast you can run the program depends on how fast you can pipe the program in from memory. Our pipe is massive -- it corresponds to sending a full feature-length movie over the internet every millisecond or so. That is how fast data comes into our system. And it can compute as fast as that".
Lead author and EECS grad student Alexander Sludds said, "In order to make something faster, you need to make it more energy efficient. But there is a trade-off. We've built a system that can operate with about a milliwatt of power but still do trillions of multiplications per second. In terms of both speed and energy efficiency, that is a gain of orders of magnitude".
The researchers tested this method by sending weights over an 86 Kilometer fiber. It resulted in ML with high accuracy i.e. 98.7% for image classification and 98.8% for digit recognition.
Story Source:
Materials provided by Massachusetts Institute of Technology.. The original text of this story is licensed under a Creative Commons License. Note: Content may be edited for style and length.
Journal Reference:
- Alexander Sludds, Saumil Bandyopadhyay, Zaijun Chen, Zhizhen Zhong, Jared Cochrane, Liane Bernstein, Darius Bunandar, P. Ben Dixon, Scott A. Hamilton, Matthew Streshinsky, Ari Novack, Tom Baehr-Jones, Michael Hochberg, Manya Ghobadi, Ryan Hamerly, Dirk Englund. Delocalized photonic deep learning on the internet’s edge. Science, 2022; 378 (6617): 270 DOI: 10.1126/science.abq8271
0 Comments