On the temporal clustering of European extreme precipitation events and its relationship to persistent and transient large-scale atmospheric drivers
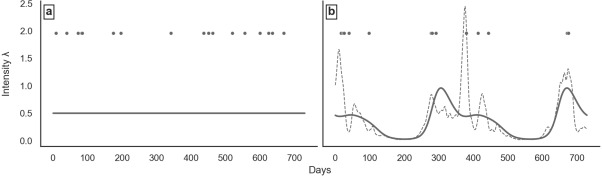
Sub-seasonal temporal clustering of extreme precipitation events (EPEs) can have profound societal and economic repercussions. First, the close succession of rainfall events can increase the time for soil moisture, rivers, and lakes to return to climatological values in catchments with high-retention capacity and cause devastating floods. Second, the short recovery time between events can overburden emergency services and prevent proper clean-up of damages and efficient repairing of damaged protective structures. Third, the temporal clustering may generate unexpected financial implications for the insurance/reinsurance industry hence, a sequence of extreme rainfall events can amplify impacts when compared with a single hazard. It is therefore a temporally compounding event. Process understanding of the temporal clustering of EPEs is needed to assess and mitigate associated risks and to improve predictability on sub-seasonal time scales.
Extratropical modes of climate variability such as the Artic Oscillation (AO), the North Atlantic Oscillation (NAO), the East Atlantic pattern (EAP), and the Scandinavian pattern (SCA) capture predominant atmospheric circulation patterns on sub-seasonal time-scales. They have been shown to govern dry/wet conditions and heavy precipitation frequency across wide parts of Europe. These modes of climate variability are further related to the position and strength of the jet stream and thereby the temporal clustering of cyclones. Modes of variability have been used to investigate if reanalyses and global climate models capture temporal clustering of heavy precipitation events across Europe. Building on the modes of variability, weather regimes (WRs) summarize regional climate variability on timescales of several weeks
The recurrent nature of weather situations is potential relevance for the understanding of the temporal clustering of EPEs. For example, synoptic-scale transient recurrent Rossby wave packets termed as RRWPs, affect persistent wet spells over the European sector, increasing wet spell duration in western Europe and western Russia during summer, and over eastern Europe and the Mediterranean in winter. How recurrent Rossby wave packets modulate the temporal clustering of individual extreme events remains to be investigated. Besides recurrent weather situations, stationary blocking anticyclones can also lead to temporal clustering of heavy precipitation as demonstrated on the Alpine south side and by selected areas around the Northern Hemisphere. Currently, systematic quantification of the link between blocks and temporal clustering of heavy precipitation in Europe is still missing.
Temporal clustering is typically analyzed using methods that assume point processes, such as the dispersion statistic Ripley’s K function or count-based approaches. In agreement with this assumption, EPEs are here defined as events lasting from one to several days consisting of independent point events along the time axis. Independence is assured by grouping consecutive extreme precipitation days into single events. Previous studies have investigated the association between the sub-seasonal temporal clustering of EPEs and the time-varying effect of large-scale atmospheric drivers in the Euro-Atlantic sector. In these studies, this association is modeled using a Cox regression model, i.e. a Poisson process with a randomly varying rate of occurrence. Such a model has also been used to relate the temporal clustering of flood events and EPEs across the central United States to modes of climate variability. Several studies have relied on Poisson regression to model sub-seasonal temporal clustering of extratropical cyclones over Europe, flood events in Austria, and heavy precipitation in the North American Midwest. However, these modeling approaches assume a linear combination of parameters and cannot parametrically account for the seasonal cycle of EPEs, which is omnipresent across Europe (Fig. 5). In a Cox regression model, seasonality is implicitly accounted for in the model through the baseline hazard, though it is not parametrically specified. In Poisson regression, seasonality can either be accounted for by including binary indicator variables for each month ( or by fitting a separate model for each season. A general additive model (GAM) lifts the linearity constraints; for example, assesses the non-linear effects of the NAO on the rate of occurrence of yearly flood counts using a Poisson GAM.
A GAM is essentially a Generalized Linear Model in which the relationships between the predictor variables and the response are specified by flexible smooth functions. The flexibility that such a model offers has many advantages over classical GLMs. A GAM does not impose any parametric form of the link between the response and the predictor variables. This flexibility allows non-linear relationships between the predictor variables, i.e. atmospheric drivers, and the response, i.e. EPE counts, to be modeled. In practical terms, it is possible to implicitly account for the seasonal cycle in EPE counts by modeling them as a smoothly varying cyclic function, e.g. a cyclic regression cubic spline, of time, i.e. the day of the year. As such, it is possible to use one parsimonious model instead of separate models for each season; parameters are better estimated and temporal discontinuities between seasons are avoided.
In this study, we propose a novel approach to investigate the sub-seasonal temporal clustering of EPEs across Europe using 42 years of daily precipitation data from ERA5. This sub-seasonal temporal clustering is defined as random spurts of activity beyond the seasonal cycle of EPE counts, which we capture through Poisson GAMs fitted using data from all seasons. Our model results provide insight into the clustering frequency over Europe, i.e. to quantify the significance of temporal clustering in EPEs and to identify unique clustering episodes. Moreover, we investigate potential links between atmospheric dynamics and temporal clustering of precipitation extremes by inspecting the effects of seven predominant year-round WRs, atmospheric blocks being implicitly captured by the WRs and RRWPs in the Euro-Atlantic sector on the temporal clustering of EPEs on sub-seasonal time-scales. More specifically, we address the following questions: (1) where do we find significant sub-seasonal temporal clustering of EPEs in Europe beyond the seasonal cycle and how is it spatially distributed? (2) If temporal clustering is detected, where and when do the atmospheric predictors modulate temporal clustering of EPEs, i.e. explain positive deviations from the seasonal cycle in EPE counts?
The paper is organized as follows: the data is introduced in Section 2. Section 3 contains methodological aspects of the EPE definition, modeling the temporal clustering using a GAM, WRs definition, and quantification of the clustering. The results and discussion are presented in Sections. Finally, general conclusions and future research avenues are presented in Section 6.
2. ERA-5 data
We use European Centre for Medium-Range Weather Forecast’s (ECMWF) latest reanalysis product, ERA-5. It is the fifth generation atmospheric reanalysis of the global climate from ECMWF and can be accessed publicly. We use precipitation fields from the ERA-5 reanalysis data set at 0.5°horizontal resolution from 1 January 1979 to 31 December 2020. ERA-5 precipitation data are available at an hourly resolution that we aggregate to daily precipitation. ERA-5 precipitation stems from short-term numerical model forecasts and is in good agreement with observational data and captures temporal clustering of precipitation well. In this study, we model the temporal clustering of EPEs at 6140 gridpoint locations across Europe in the domain 30N-72N and 25W-40E. We use the ERA-5 land-sea mask to restrict the analysis only to land locations for computational efficiency and relevance in terms of impact. For convenience, we omit February 29 from all leap years contained in the 42 years of data.
Methods
Modeling the temporal clustering of extreme precipitation events
Definition of extreme precipitation events
We apply a Peak Over Threshold approach to select extreme rainfall days. The threshold for extreme rainfall days is the all-day 98th percentile of the local daily precipitation accumulation at each gridpoint (Fig. 1a). This threshold is chosen based on a trade-off between a high enough precipitation level and retaining enough data. An extreme precipitation event is defined as an independent point or occurrence along the time axis and therefore runs of consecutive exceedances, i.e. extreme rainfall days, are declustered. We apply runs declustering with a run length of 2 days, i.e. exceedances separated by no more than one day belonging to the same event. A run length of 2 days has been determined based on objective statistical methods about the extremal index and corresponds to the average time that the same mid-latitude weather system would generate extreme precipitation at a given location. For events lasting longer than one day, the date of occurrence of the event corresponds to the day of the precipitation maximum within the event. The total number of independent EPEs ranges from 202 to 301 across the study domain (Fig. 1b).
Story Source:
Materials provided by Weather and Climate Extremes. The original text of this story is licensed under a Creative Commons License. Note: Content may be edited for style and length.
Journal Reference: Science direct
0 Comments